Next: Thermodynamic Forces & Kinetic
Up: Time Dependent Fluctuations
Previous: Time Dependent Fluctuations
Subsections
Suppose we have a thermodynamic variable x with
. What
happens if at some moment
?
- Thermodynamics Language:
- We have a restricted ensemble:
a set of systems with the given x.
- Example:
- I prepared a set of beakers at the temperature T0
and put it in the thermostat with the temperature T.
- Kinetics and Equilibrium:
- A beaker at the temperature T is
not in the equilibrium with the environment--technically
speaking, we cannot use thermodynamics!
- A Trick:
- we say that it is partially
equilibrated--everything is equilibrated for this
temperature.
- General situation:
- we have a slowly changing variable x, and
say, that every other degree of freedom is fast.
Then we have a function
, such as
|  |
(1) |
At
we have
(why?). For
small deviations
is small. We can expand it in
series in
, and
| 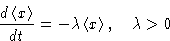 |
(2) |
Solution:
|  |
(3) |
Small fluctuations decay exponentially with the relaxation time
.
This formula is valid only for t>0: we can prepare an ensemble
with given x0 and observe it, but cannot go backward! This is
another manifestation of time irreversibility.
For space-dependent problems we defined the correlation
function
. For time-dependent case we define
time correlation function
. We want
to calculate
. This is the averaging over the ensemble of
systems with arbitrary x(0). Note this difference with the previous
case: there we had x(0) fixed, and used restricted ensembles.
Now we return to the general case and consider equilibrium
ensemble.
Translation along the time axis:

and therefore
|  |
(4) |
Double averaging method:
- 1.
- Divide the ensemble into subensembles with given
x(0)=xi. Each subensemble has many microscopic states with
given macroscopic value x(0).
- 2.
- Average x(0)x(t) in a subensemble:

- 3.
- Average
over all subensembles:
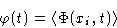
Since in a subensemble x(0) is given,

Second averaging:

with generalized
susceptibility
.
We obtained for the correlation function:
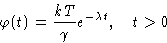
From (4) we obtain for negative t that
, or
| 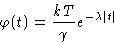 |
(5) |
This function has different derivatives at
: time
irreversibility!
We have several variables xi. Kinetic equations:
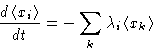
Now we have multi-variate correlation functions:

- 1.
- Translation of the time axis:

We see:
| 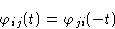 |
(6) |
- 2.
- Equations of motion are symmetric with respect to time inversion
:
| 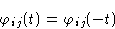 |
(7) |
- 3.
- From (6) and (7)
| 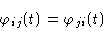 |
(8) |
Next: Thermodynamic Forces & Kinetic
Up: Time Dependent Fluctuations
Previous: Time Dependent Fluctuations
© 1997
Boris Veytsman
and Michael Kotelyanskii
Tue Oct 28 22:13:33 EST 1997